Why Fragmented Security Solutions Often Fail to Protect Your Data
0 分钟阅读
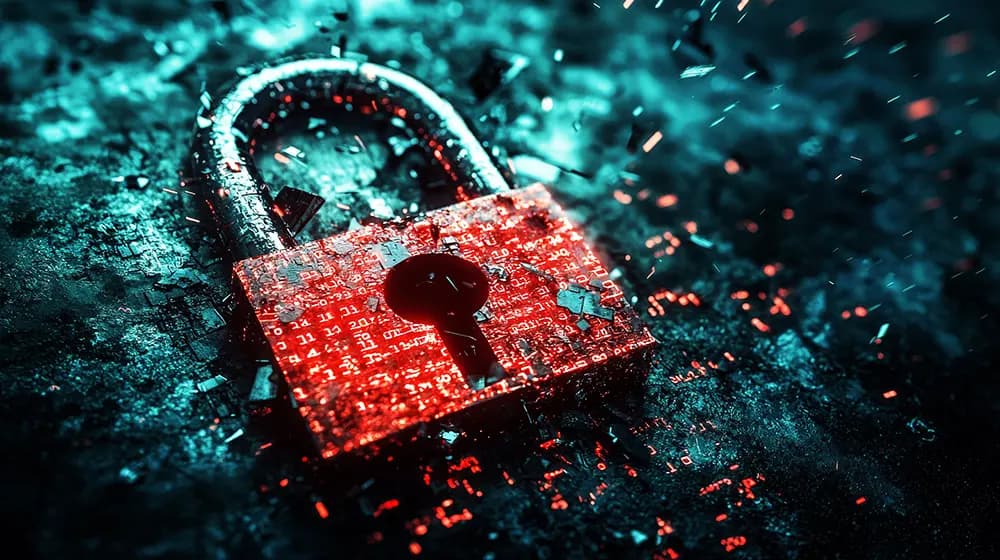
Data security comes down to visibility and control. Visibility means being able to detect sensitive information, which we can break down into regulatory compliance data and intellectual property. A mature ability to identify both regulated data and intellectual property with low false positives is the first data security challenge many organizations face.
Subpar solutions may have a few out-of-the-box templates for a handful of regulatory compliance mandates, but they often lack advanced capabilities needed to control intellectual property or other sensitive data. They also have high rates of false positives, i.e. they incorrectly classify data as sensitive when it is not, which can add friction for users and slow down productivity.
Yet another challenge is implementing this visibility consistently everywhere sensitive data is used. This requires controls to be implemented on endpoints, across email, the web and SaaS applications. And finally, we need to decide when to go from auditing into blocking mode, and, more importantly, how to do it seamlessly. This usually is tied to another question: since sensitive data is used so often in so many ways to drive the business, how do we effectively prioritize potential data exfiltration events and how do we scale this across the organization without adding friction and slowing productivity?
How a fragmented security model limits data protection
First, let’s look at the implications of using a fragmented security model, a problem which is far too common today. A data security model can become fragmented in many ways. One depends on the types of data a solution is good at detecting. If a data protection tool can only identify PII and PCI data effectively, that means you are blind to intellectual property—the most valuable type of sensitive data in an organization, whether that is source code, pharmaceutical formulas, schematics, etc. IP represents the ‘secret sauce’ that makes each company value to customers they serve. Protecting your company’s crown jewels is paramount.
Another data security challenge is consistent visibility over all major channels, which often means organizations have different solutions for different channels with some channels completely unprotected by data security policies. This often results in blindness and wide-open data security gaps where there are no controls; It also means different views of data security risk for each channel covered by a different product
This complexity often results in paralysis where systems don’t agree whether a certain piece of data fits a classifier or not (i.e. controls on the endpoint may be more accurate while controls on the web could have higher false positives), all of which can lead to security gaps or unnecessary friction for end users regardless of how the organization deals with the differences. All of this points to some of the hardest challenges:
What are the right ways to control the movement of sensitive data, when should we block this movement, and once we hone in on an effective data protection strategy and policies for our first set of test users, how do we scale this?
With a fragmented security model, many organizations never get past the ‘audit’ phase, which represents the most basic level of control. That relegates them to simply just keeping a log of what data moves where and does nothing to stop sensitive data loss. Even if an organization has the capability to accurately identify sensitive data types across most data exfiltration channels, distinguishing between which actions are truly risky, versus standard user behavior can be a very difficult challenge that ultimately ends up in the organization having to stop using ‘blocking’ controls and revert to audit only. This issue acts like the inverse of fragmentation—the system can’t understand the context of an event so all alerts are equal.
A unified approach to your data protection strategy
Now, let’s examine how everything changes with a unified data protection strategy. When we deploy an industry-leading Data Loss Prevention (DLP) solution at the heart of a unified platform that uses advanced classifiers with exact data matching, machine learning and AI-powered classification, we can instantly clear the first hurdle of accuracy. As you evaluate solutions, it makes sense to test against the data types your organization uses and also to assess false-positive rates. An ideal solution will result in low false-positives for the data that is most important to you.
Next, using a cloud platform that natively extends best-of-breed DLP policies to web and SaaS applications, while also providing support for email and deep endpoint controls ensure consistent visibility and control across those channels. This is further strengthened by a platform that adds context by dynamically assessing user risk, empowering you to overcome the challenge of prioritizing the most meaningful data security events. This approach enables you to set different enforcement controls by risk level, allowing minor infractions to occur without introducing too much friction, while allowing for increased controls as users exhibit more blatant risky or malicious behavior, unlocking the ability to easily scale such a solution across the entire organization.
To learn more about how Forcepoint Data Security Cloud platform helps seamlessly protect your organization’s data, talk to an expert today.
Corey Kiesewetter
阅读更多文章 Corey KiesewetterCorey Kiesewetter is Forcepoint’s Sr. Product Manager for cloud security products, with a focus on data security and Zero Trust. Corey has been directly helping IT practitioners realize best practices in datacenter operations the past decade and holds a degree in Philosophy from the University of Texas.
- The Forcepoint Data Security Cloud PlatformLearn More
X-Labs
Get insight, analysis & news straight to your inbox
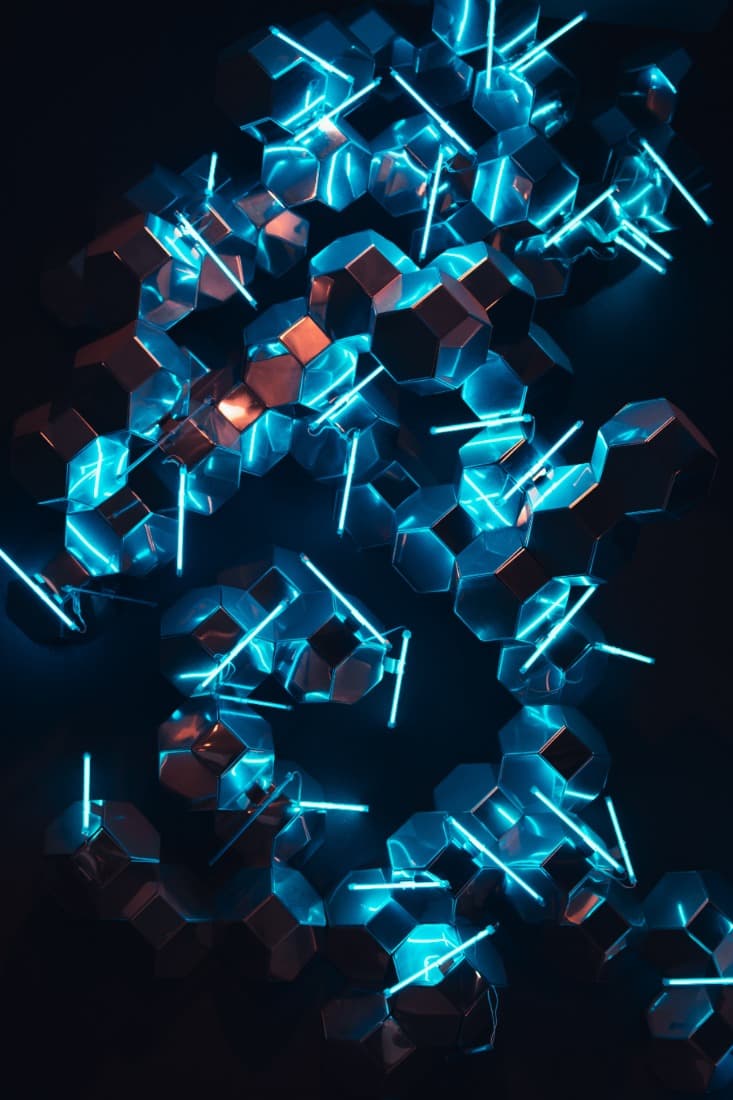