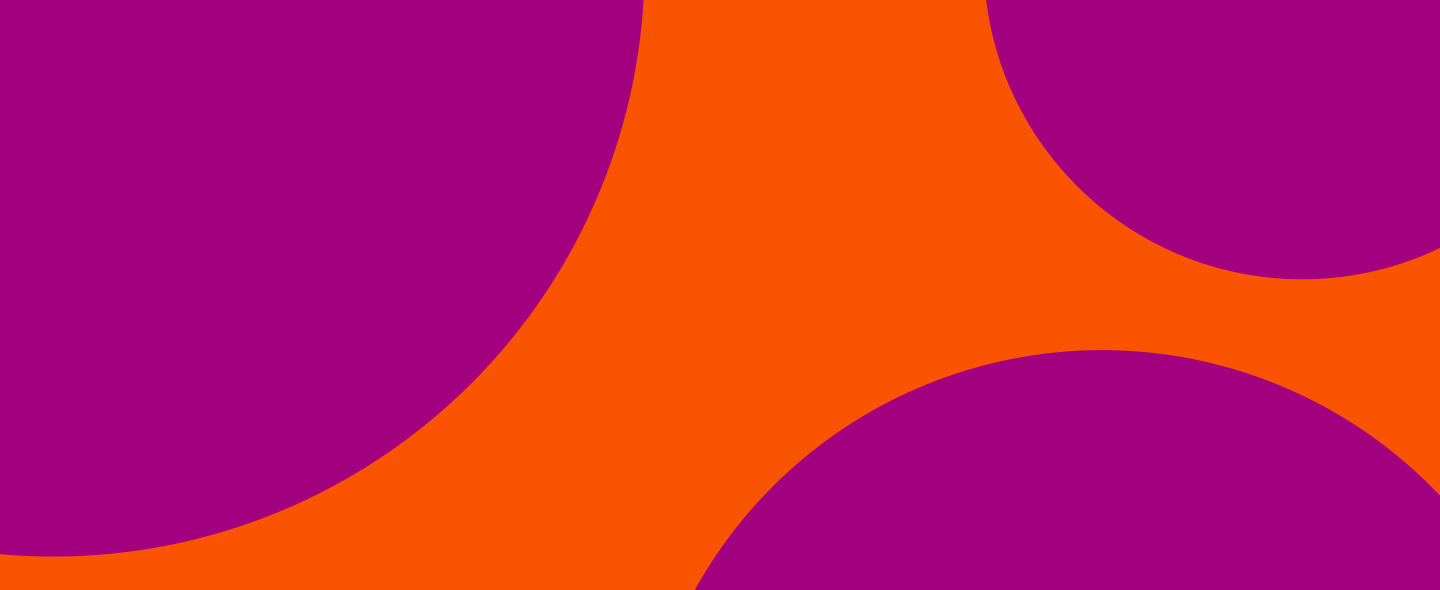
Navigating Today’s Insider Threat Through Human-Centric Behavior Analytics, With Toby Ryan
Share
Podcast
Popular Episodes
50 mins
REPLAY: Someone Needs to Do Something, But Who?
Episode 278
March 26, 2024
47 mins
Cyberwar, Social Media’s Future and Passing the Mic with Peter W. Singer
Episode 206
November 8, 2022
56 mins
The Conga Line of Cybersecurity in 2022 with Manny Rivelo
Episode 167
January 25, 2022
48 mins
See Something, Do Something: A Conversation with Dmitri Alperovitch
Episode 160
November 30, 2021
Podcast
Navigating Today’s Insider Threat Through Human-Centric Behavior Analytics, With Toby Ryan
For organizations, managing insider threat is a journey, as they steadily build up their capabilities to effectively mitigate risk. Toby Ryan, VP of Analytics Engineering at Forcepoint, discusses how to develop a smarter, more comprehensive approach that combines business and compliance processes so that the level of protection keeps pace with an ever-expanding and evolving digital threat space.
Don’t forget to sign up for upcoming episode alerts!
Episode Table of Contents
- [3:33] The Detection Methodology
- [5:04] Detecting Through a Pattern of Behavior
- [7:43] Understanding the Detection Value of Your Data
- [10:41] The Fear of Missing Something
- [13:49] The Equation to Optimize
- [15:36] What is Machine Learning?
- [24:01] We Can See Signs But We Can't Predict
Introducing Our Guest, Toby Ryan
Arika: We have a great guest today. We have Toby Ryan of Forcepoint who is the VP of analytics ... engineering. Sorry about that. How are you doing, Toby? And thanks for being on the podcast.
Toby: Good. How are you? Thanks for having me.
Arika: Good.
Eric: Toby is the scientist of scientists in the organization, really making the products do what crazy people, including Tony ... Toby, excuse me, think up, dream up as the art of the possible. He and his team deliver the capability. It's great.
Arika: Well, I was going to say with a title like that, obviously you're very smart, so we're excited to have this conversation with you today and learn a little bit more. Let's start here. We always do a little prep before the podcast, and we were talking about, Toby, how a lot of your focus is building technology and understanding how it interacts just with the human mind. I know it's been a common theme that we've had throughout the podcast.
Arika: Anyone who heard a few episodes ago, maybe about, I don't know, six or seven episodes ago, I was talking about how I actually clicked on a phishing email, even though I cohost a cybersecurity podcast.
Eric: Oh, we're going back to that again?
Toby: Which one?
Eric: Which one?
If It Looks Different, Don't Click It
Arika: No need for the details, but and it's funny because something came up at the organization that I work with most recently where we were changing the marketing platform for our internal emails, and a couple of folks within the organization were hesitant to click on them because they said, "We've been taught that when it looks different than what we've seen, we should not click."
Toby: Good.
Arika: It's funny ... which is good, right? It means the training is working, but now we're trying to actually tell them, "But we want you to click, even though it looks different." It's trying to figure out how do you, I don't know, change that mindset, but also keep the right mindset, especially when you're thinking about it from a technology and security standpoint?
Arika: Toby, that was my long way of asking you, how do you approach those types of things, especially when it comes to just your very basic phishing emails, which we've all seen? As humans, some of us, I'm sure it's maybe a 50/50, don't know the percentage, that we want to click, some of us are hesitant to click, how do you navigate that especially when you're thinking about new products and services to enhance security?
Toby: Yeah, absolutely. It's interesting that you bring up phishing as an example. I think a lot of the major reports out there on the state of cybersecurity show that fishing is still absolutely the number one threat and intrusion factor.
Eric: Because it works.
The Detection Methodology
Toby: The fact ... Yeah it works, and it's well over 90% if you average most of those reports together. Nine out of every 10 attacks start with spear phishing or phishing because it works. But yet, conversely, there is a huge part of the cybersecurity industry that's devoted to stopping phishing through edge-type defensive devices. If you think about the path of a phishing email, it has to go through firewalls; it has to go through web security; it has to go through email [AV proxy 00:04:06], all kinds of things. [Crosstalk 00:04:08].
Eric: We call this millions of dollars of product, right?
Toby: Absolutely, and yet, it still gets through and advanced persistent threats are very, very good at it. The idea is how do you detect that? Well, you have to look at the behavior of what happens when you click on a phishing email, the ... if you remember back in the eighties and the nineties, the detection methodology was very signature-based. Hey, if you see A and A is bad, then fire an alert.
Toby: We've moved forward to the point where we understand that both humans and devices exhibit behavior, and in order for a phishing email to be successful ... Let's say that you're an enterprise user of Outlook ... like most organizations are ... Outlook has to do something. Malware doesn't magically execute, so Outlook has to open something. Well, if Outlook spawns PowerShell, that's usually really bad, right?
Detecting Through a Pattern of Behavior
Toby: But if Outlook opens up Adobe because there's a PDF attachment, that's fairly normal, but the behavior of malware is very distinct, and you can't detect that through one signature. It's a pattern of, first of all, what looks normal, right? When you click ... we don't want an alert every time someone clicks on a PDF. If someone clicks on a PDF, and Adobe spins up and it does something, that's very normal, but if you click on that PDF and Adobe spawns PowerShell, that's a problem. That's not really Adobe.
Toby: You detect that through a pattern of behavior, but you have to know what the pattern of normal looks like. What's interesting about that is that's not a statistical shot in the dark or some kind of machine learning. It's actually quite, quite basic. It's this is what a computer does when you click an attachment. When you understand what happens when you click on an attachment, you're able to understand what's normal and what's not.
Toby: You can use some of those methods as supporting detection methodologies. For example, if it spawns a process you've never seen before in your environment, that's an outlier. That weights it more heavily, but at the very core of it, it's actually much simpler than I think the cybersecurity industry makes it out to be.
Chain the Patterns Together
Eric: Toby, if I'm a developer, I'm using PowerShell quite frequently. What you're saying here is it's really the application Outlook that gives you the tipper, if you will, that something's probably not right.
Toby: Yeah, absolutely. Again, that comes from ... and I think that's one thing that I like a lot about Forcepoint is myself and a lot of people on my team are dedicated to understanding what ... Our company motto, free the good stopped the bad. We don't want to get into the scenario where we're alerting every time a developer uses PowerShell. That's the old school like we're just going to create this engine that finds bad stuff, and we don't necessarily care about delineating how bad or false positive. I have-
Eric: It's basic rule-based analysis, right?
Toby: Exactly.
Eric: If X happens, do Y or alert or whatever behavior.
Toby: Exactly.
Eric: But it's really dumb logic.
Toby: It is and the idea is to chain these patterns together. I have researchers working on this. We send emails to each other with attachments that aren't malware, but they exhibit different behaviors so we can test and understand what normal looks like. We had-
Arika: Oh, really? That's interesting to know. Okay.
Understanding the Detection Value of Your Data
Toby: Oh, absolutely. Yeah, no, that's the best way to do these things. The idea is if a developer's using PowerShell, we have to understand how often and how regularly and what they're using it for. Well, to do that, we have to have an understanding of what PowerShell is and does. It's a built-in Windows tool that administrators often take advantage of and Microsoft promotes heavily for a good reason.
Toby: However, it's also one of the number one, what they call LOL attacks, living off the land, where hackers use tools that come with Windows to ... There's no need to write custom malware when you can use what Windows already gives you. [Crosstalk 00:08:27].
Eric: Well, they know it'll be there, so they know they can leverage it.
Toby: Absolutely.
Eric: It's free.
Toby: We have to delineate between normal PowerShell usage and abnormal. Well, how do you do that? That really comes back to understanding the detection value of your data. You can throw in a thousand data sources, but understanding what in those data sources and what data sources in general lead to higher quality detection is the most important thing. They're only a finite number of PowerShell logs that will give you the information you need, and they're incredibly noisy, so you have to further get that to a smaller number for this to be effective.
The Fear of the Manual
Toby: That's work that is really understanding the problem, and that's a work that I find that if you call cybersecurity part of IT, there's the fear of the manual. No one wants to really dig in and understand behavior, understand intent, and yet that's what's required. I could build a dumb statistical engine that tells you every time PowerShell is used, but then that's just going to cause analysts to have to deal with a lot of false positives.
Eric: Well, you might as well just remove PowerShell from the environment, right?
Toby: Right and that's not, of course, going to go over well at any enterprise because most use it, legitimately.
Eric: Well, let me ask you-
Arika: [Crosstalk 00:09:52] find a workaround, right? That's what we've talked about before is that when you do things like that, then people will always find a way to work around it, which is not good for the environment as well.
Toby: Exactly.
Eric: Toby, when I meet with customers, especially around these topics, the most frequent request I get is, "How many data sources can you collect from?" I have a ton of data in my data lake. I look at it more like a dumpster, but that's okay. "We have massive amounts of data. I need someone to help me sort through it." I mean, I hear this over and over and over again. The problem I tend to have is it's really difficult to get IT or security professionals to articulate what behaviors or problems they're trying to identify and stop.
The Fear of Missing Something
Eric: What problem are you trying to solve for here? Because many times from my work with you and the team, it could be a simple rule or two datasets. You had two data sources where we can clearly tie into whatever they're trying to accomplish. Yet, they want to feed 85 data sources into the system and just throw it all in there and somehow magically shake it up like an eight ball and have the answer come out. Why is that?
Toby: I think there's a couple of reasons for it, and there's probably the main reason is the fear of missing something. You want to put in as much as you can, thinking that sheer quantity will solve your problem. I think the other part of it is understanding. I mean this gets back to almost what I was just talking about is that in order to understand the value of your data, you have to look at it piece by piece, field by field.
Toby: That's an intensive process, and as a former incident responder, that's what we had to do is we had to dig through logs and understand what these things meant. I don't think a lot of companies really know what's in that data, and that's where we come in is, okay, let's say you're giving me Windows event security logs. [Crosstalk 00:12:05].
Eric: I'll always get a request for that, that and feed all email into [inaudible 00:12:07].
Toby: Yeah, absolutely.
Eric: As their basics.
There Are a Lot of Things We Can Do
Toby: Right. It's one of the most verbose logs out there, especially if you combine the host base with the network base from the domain controllers. You get millions and millions of logs per hour. Well, there's a lot of things we can do. There are certain Windows events and security codes that are more valuable than others. Even within an individual log, which could be anywhere from 15 fields to 50 fields. Even within that log, there are only certain maybe five or 10 fields that we actually need.
Toby: We can get rid of a lot of this data that's not contributing to detections, and it makes everything more efficient, faster systems, less hardware, all done in a productized pipeline when you bring in this data. But the idea is to say, "Okay, for this data source, what can I detect?" There are two ways to approach this, and I don't know really which one I prefer more. They're both valuable for different reasons, but you can either say, "Here's data source A, Windows event security logs. What can I detect from this data source?" Well, I can look at a lot of [past the hatch 00:13:19] lateral movement, first-time logins to new servers, all kinds of interesting things.
Toby: You can tie a lot of those together and chain them into detections. Or you can start with, "I'd like to detect lateral movement in my environment. How would I do that?" Well, you could use Windows event security logs. Whether you start with the use case and get down to the data source you need or you start with the data source and move up to the use cases you want to solve for, either a way gets you to the detection value of the data.
The Equation to Optimize
Toby: Because even within ... let's just say that there are well over a hundred to 200 Windows event security codes. Maybe only 10 or 15 of those contribute to a lateral movement pattern, and also whether you're getting them from the host or from the domain controllers matters a lot. I would say don't throw in a thousand data sources. One, I mean that's ultimately what a lot of what my team does is understanding this detection value. But the more you understand about it ... because you're going to pay for all that compute. Compute is not free, even in the cloud.
Toby: You can't just throw in a thousand sources and expect to ... I think, we were talking earlier about this, Eric, about the ROI on detection science and what value are you getting of all of those logs for the detections that are coming out of it. That's the equation that I'm trying to optimize.
Eric: Yeah, it's interesting. You and I've in the past. One of the main things that stuck out in our discussions in my mind at least was there are a couple of components that really determine which indicator or detection methodology you use, or when you choose to use it, and it's really mathematical complexity as I recall, fidelity of the data source, and then the difficulty level of implementing. That really opened my eyes. We talk about machine learning; we talk about artificial intelligence, but some of that's really hard and really not needed depending on what we're trying to solve for.
Toby: Yeah, no, absolutely. You hit on my soft spot there. As a-
Eric: Mine too now, I guess.
What is Machine Learning?
Toby: Right?. As a data scientist by trade, it makes me cringe when I see all the hype surrounding machine learning. It's valuable as a supporting cast member. It's valuable in aiding towards detections, but we're not yet advanced enough as an industry to just throw stuff at machine learning and expect to have high-fidelity answers come out. The very definition of machine learning is function approximation. Mathematically, it's function approximation, and the keyword in there, if you know nothing about math, is approximation.
Toby: If you're telling an analyst, "Hey, maybe this is bad; maybe it's not," you're not making that analyst very happy. You have to look at using all of the methodologies available. You can detect some wonderful things with simple rules. If a new ransomware [inaudible 00:16:33] comes out, you just take that [inaudible 00:16:35] and make sure you're not seeing it anywhere.
Toby: That's you know, AV at its basic self, and there's nothing wrong with that. As you move up the continuum, you start looking at pattern recognition, like we talked about in the phishing example. Clicking on an email attachment is perfectly good. However, what that attachment does after that, that determines whether or not it's malicious moving forward. We don't want to fire on anything until we know it's pretty much malicious.
The Difficulty in Getting Anomaly Detection Techniques Right
Toby: As you move up and you get into things like anomaly detection or novelty detection, those are methodologies based on certain types of statistical distributions of which you hardly ever find in an enterprise dumpster fire environment. For all the analysts out there, they know what I'm talking about when they look and something is supposed to be normal, and yet it's completely not, and something that's supposed to not be normal is completely normal.
Toby: There is no way that an enterprise network exhibits any type of normal behavior, and so using a lot of those anomaly detection techniques are very, very difficult to get right. They're very easy to implement; they're just very difficult to get right, so you want to use it appropriately and pragmatically. That's the problem is I want to solve for the 95% that I can catch and see with a medium level of complexity, and then I'm going to move to that 5%. I think as an industry, I think everyone's chasing the 5%, the shiny AI machine learning part.
Eric: Arika, that's all we hear about. Right? Artificial intelligence and learning.
We Can't Leave the Machine Figure Things Out
Arika: Well, yeah. It's funny because that was my next question, and I know we don't have too much time left, was you said that we're not advanced enough to throw everything at machine learning or AI, and we've had a lot of episodes on that. But do you see that though in the future being a true component of the security industry?
Arika: Or do you think it's to your point that we should just really stick to applying these simple rules because you'll never be able to get I guess to the place where you could truly have by probably what's the wrong definition, given that you just gave us the true one, of what machine learning and AI is supposed to in theory do or what it aspires to do?
Toby: Yeah. I think we're going to get better at it, and I think we're going to get better at it by looking at it and realizing what it is and acknowledging that it's a supporting cast member in reality. It will give you very good answers. It's just whether or not those answers stand alone by themselves as being sufficient to call out a detection as something that's a true detection.
Toby: I think I certainly love the application of machine learning as a contributing observable into a detection pattern, but I can't think of anything that we have that says we're just going to leave it to the machine to figure this out and tell us yes or no.
Expectation Versus Reality
Eric: When you say "we," you mean the industry?
Toby: Yeah, the industry. Absolutely. Yes.
Eric: There you go. We just feed it in and get the right answers back.
Toby: Yeah, and I think what you saw at the beginning of this hype about five to eight years ago in that timeframe when the hype was really starting to enter the hype cycle was that was the expectation, and that's just not reality. Now, the reality is it's very important. I don't want to go back to the early nineties where it's just simple rules. I want to combine the best of all worlds and say, "Hey, I have simple rule A, complex rule B, and machine learning classification C.
Toby: I want to take A, B, and C and make detection D. Now that is absolutely where I think the future is. That's certainly what we're trying to do. I feel that's cutting edge. It's taking all of the best things. By the way, that covers a lot of the data sources that you see in the cybersecurity world. You can get those things from all those data sources. I think that's where it's going, and it's not that the focus is on AI machine learning. It's the application and how it relates to a greater cybersecurity detection. I think it's good science. I just think it's being misused.
Eric: It's amazing because all of my customers are talking about AI, machine learning. That's where they're spending their money. That's the solution for the future. Like you, Toby. I was in the military. It was in the Army. I keep going back to the KISS principle, I was in infantry. I don't know.
Arika: But-
Replicating the Human Brain
Eric: Keep it simple [inaudible 00:21:16] has served me so well over a lifetime. We aren't even getting basics right in this industry.
Arika: I think everyone's watching a lot of Black Mirror, so we just want the answer to everything to be a machine learning.
Eric: You think that's it?
Arika: Look, even our emails now on G-mail are using things like machine learning, so I think it's what people ... Everyone thinks across every industry it's the answer. I believe so.
Eric: I don't know. I feel like as soon as the machine learns something or yeah, I don't know, the adversary shifts and all of a sudden the models have to change. It has to be able to react, and there's a lag factor.
Toby: And so what-
Eric: Even in the best of models that I've seen in practice. We're not there yet.
Toby: If you think about it, what's interesting is we go back to the genesis of AI, up at Dartmouth College, people like [inaudible 00:22:10] get together. How do we make a machine think like a human? That's been the challenge ever since that happened. Until you can replicate completely the mind of an attacker or an insider threat, and I don't think you're going to be able to achieve what the true definition of AI is. I think we'll get part of the way there with some machine learning techniques, but the challenge has always been to replicate the human brain, and I think we all know how hard that is.
Human AND Machine Learning Algorithm
Toby: You just said it. When the adversary changes tactics, is the algorithm smart enough to change with it? Now I'm a huge proponent of a human coming in and interacting with a machine learning algorithm to make it better-
Eric: Agreed.
Toby: ... like a reinforcement kind of way. Now that's great. Now that's very powerful, right? But if you just expect the machine to do it, it's not going to happen.
Eric: Arika, a question for you. You're a semi-complex individual. Do you think a machine's ever going to figure out what you're going to do next?
Arika: Ah, good question.
Eric: Would that be a good thing?
Arika: Well, sometimes I don't know. Well, I'll be honest. I am someone that actually does watch a lot of Black Mirror, and I do think those scenarios where you see the ability for a machine to anticipate your thinking, where you're going, taking something that's on your mind and you automatically have ordered it from Amazon without having to open your computer and things like that. I mean I think it sounds a little scary, but it's an interesting concept. But we'll see.
Arika: Look, who would have thought that we would have been using things like Uber 20 years ago? You never know what we may rely on machines for 20 years from now.
We Can See Signs But We Can't Predict
Eric: You know, I'm always taken back to a conversation I had with Steve Grobman, CTO at McAfee. We were talking about the difference in machine learning and how scientists can predict where hurricanes are going to land with relatively high accuracy. You take a different type of meteorological event though like an earthquake, and despite the best of science today, we still can't predict. I mean we can see signs; we know that activity is happening, but we don't know when that earthquake is going to strike, and more importantly, where it's going to strike, unlike a hurricane.
Eric: There are certain things where the mathematics, the models, the science can absolutely apply.
Eric: But I think there are many others where they're as complex as you and I, Arika, and I don't know.
Arika: Not quite.
Eric: What are we having for dinner tonight? I don't know that the machine can predict that [crosstalk 00:24:48].
Arika: Hey, I'll take it. If a machine can predict it and cook it and deliver it and so ...
Eric: I'd love to just have it make it, right?
Arika: Exactly.
Subscribe to To the Point Cybersecurity on Apple Podcast and Give Us a Rating
Arika: Well, Toby, thank you so much for being on the podcast. This was quite a ... It was a very insightful conversation, and I think you've given us a lot to think about, especially as we do think to do and look to the future, especially it's this reflecting time of year. Thank you very much.
Toby: Yeah, of course. Anytime. Thank you for having me.
Arika: Well and thanks to all our listeners out there. Please continue to tune in every week to To The Point Cybersecurity, subscribe on iTunes or whatever podcast platform is your choice, and give us a rating. Also, let us know what you want to hear us talk about. Until next time, thank you.